Why Do Face and Document Verification Models Fail in Africa?
Navid Scheybani
Chief Growth Officer
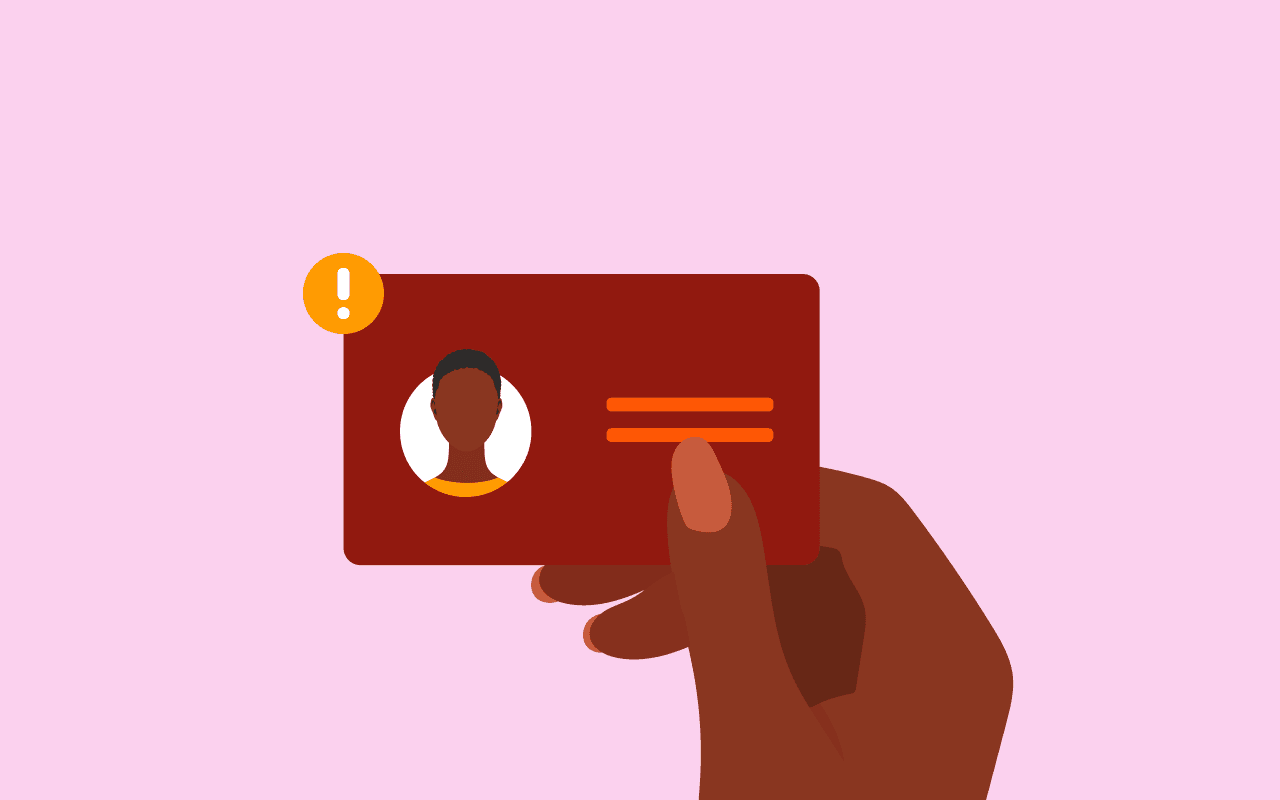
Face and Document verification models are computer systems that use facial recognition technology to authenticate a person's identity based on their facial features. These models are widely used for various applications, such as security, banking, social media, and e-commerce. However, many face and document verification models are plagued with racial biases, i.e. they perform worse for some groups of people than others based on their skin tone or ethnicity.
Most face and document verification models fail in Africa. According to a study conducted recently, global face models have an accuracy of 10 to 100 times lower for African faces.
Why are most models biased?
The lack of data representation is the primary reason for face and document verification model bias. Machine Learning models are only as good as the data they’re trained with. Unfortunately, most datasets used to train face verification models are from the global west or regions with predominantly Caucasian populations. One study by Buolamwini and Gebru (2018) showed that 77.5% of the images in three popular face recognition benchmarks (IJB-A, Adience, and LFW) are of individuals with light skin tones, while only 4.4% are of individuals with dark skin tones.
This imbalance in data representation ignores that African populations have the highest level of phenotypic variations worldwide due to their long evolutionary history and diverse environmental adaptations (Tishkoff et al., 2014). Phenotypic variations refer to the observable differences in physical traits among individuals of the same species. These variations can affect facial features such as shape, size, colour, texture, hair type, eye colour, nose shape, lip shape, etc.
The second leading factor for this bias is the lack of cultural awareness and sensitivity. Many face and document verification models assume that certain features or formats are universal or standardised across different regions or countries. For example, some models may expect a passport photo to have a plain white background, a frontal pose, and a neutral expression. However, these requirements may not apply to passports from African countries, where different colours, poses, expressions, head coverings, or accessories may be allowed.
What are the consequences of bias in face and document verification models?
The low accuracy of face and document verification models in Africa has severe consequences for businesses that require customer verification before they can offer services, e.g. financial services. Some of the potential implications of this bias include the following:
- Customers may be subjected to unfair treatment or discrimination by authorities or institutions that use face or document verification for identification or screening purposes.
- Customers may be denied access to online services or platforms that require face or document verification for authentication or verification purposes.
- Customers may ultimately lose trust in technology providers or developers who fail to deliver accurate and reliable face or document verification solutions.
- Businesses may lose customers, revenue, or reputation when they fail to provide reliable and secure identity verification solutions.
- And ultimately, the lack of accurate face and document verification models in Africa may hinder digital inclusion and innovation.
How can we overcome this challenge and build more fair and robust face and document verification models for Africa?
One possible solution is to use more balanced and representative datasets that capture the diversity of African faces. For example, creating a focused dataset of representative faces that helps train face and document verification models for otherwise underrepresented demographics that achieve higher accuracy for African faces. This is one of several approaches Smile Identity has taken to create unbiased models with the help of several million verified African faces from 15+ countries across the continent.
Another possible solution is to use post-processing methods that calibrate the fairness of face and document verification models without retraining them. For example, FairCal is a method that adjusts the decision thresholds of the pre-trained face and document verification models based on their performance on different groups of people. This method can reduce the racial bias of face and document verification models by up to 80% without sacrificing accuracy.
However, these solutions are insufficient if we do not consider other factors affecting the quality and reliability of face and document verification models in Africa. For instance, we must account for the challenges posed by low-quality cameras or internet connections on mobile devices. We must also ensure that our face verification models are robust against spoofing attacks or fraud attempts using photos or videos. Finally, we need to respect the privacy and consent of our users when collecting and processing their biometric data.
To address these factors, Smile Identity has developed a data-centric approach focused on improving face recognition's data collection and processing stages. Specifically:
- Using its large-scale dataset, Smile Identity has trained its own proprietary deep learning model specifically with African faces.
- Smile Identity has optimised its model for mobile devices by reducing its size and complexity without compromising its accuracy or speed.
- Smile Identity has implemented anti-spoofing techniques like liveness detection or motion analysis to prevent fraudsters from using fake images or videos.
- Smile Identity has integrated its model with multiple sources of identity information, such as national ID databases or biometric registries, to provide comprehensive identity verification solutions.
- Smile Identity has followed ethical principles such as transparency or accountability when collecting and processing user data.
By doing so, Smile Identity has achieved over 99% accuracy for African faces across different scenarios and environments. It has also built unbiased algorithms that do not discriminate against any group of people based on their skin tone or ethnicity.
In conclusion, most face and document verification models are biased against African faces because they are trained on datasets that do not reflect the reality and diversity of African people. This bias leads to poor user experience and business outcomes for African users and businesses.
To mitigate this bias, we need to use more balanced and representative datasets and post-processing methods that calibrate the fairness of face and document verification models. We also need to consider other factors that affect the quality and reliability of face and document verification models in Africa, such as device limitations, spoofing attacks, or privacy concerns. Doing so can improve the quality and reliability of identity verification services in Africa and foster digital inclusion and innovation in the region.
Ready to get started?
We are equipped to help you level up your KYC/AML compliance stack. Our team is ready to understand your needs, answer questions, and set up your account.