Dynamic Liveness Detection: The Most Secure Way to Onboard & Authenticate Users in 2025?
Emmanuel Agwu
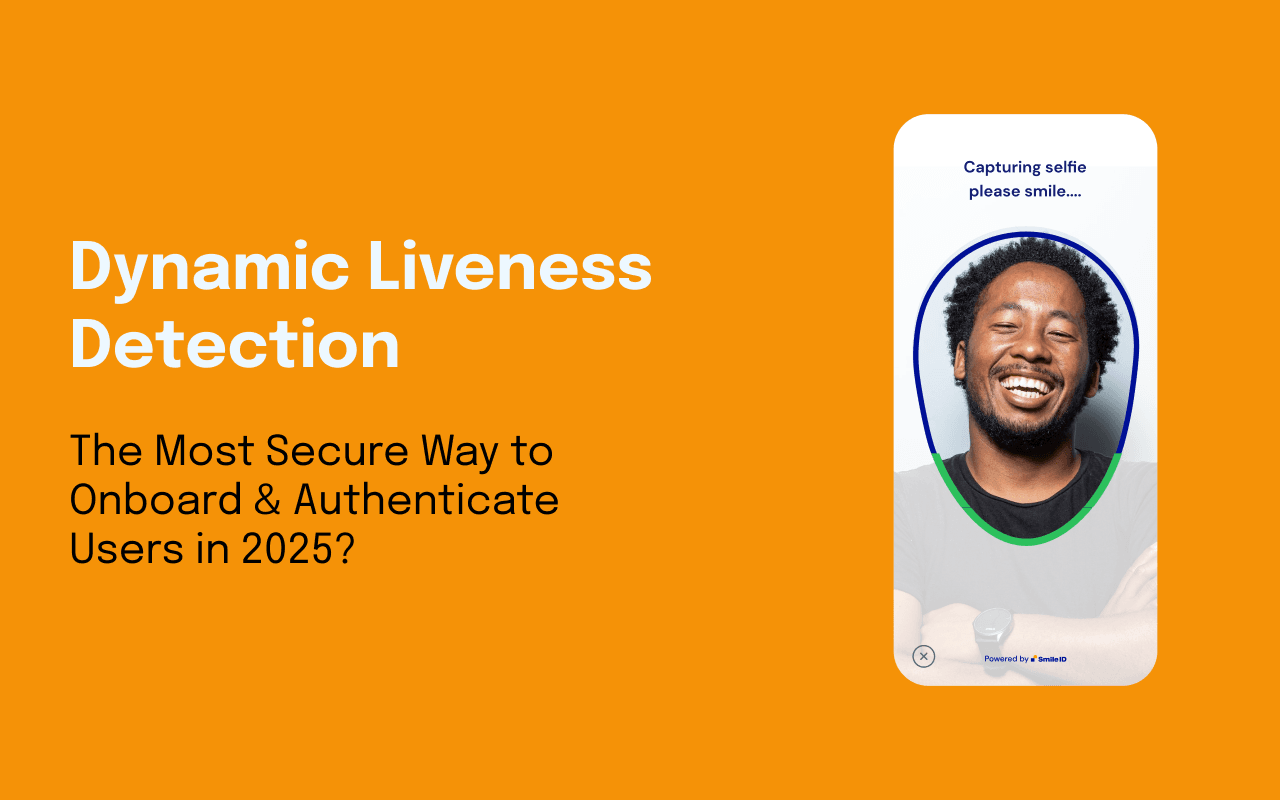
Today, identity fraud losses are estimated to stand at $5.4 trillion globally, with digital onboarding channels as prime targets for fraudulent attacks. Traditional identity verification methods—like static passwords, OTPs, and document scans—have been insufficient to combat these increasingly sophisticated threats. With fraud rates projected to climb further, organisations across industries are urgently seeking robust solutions to protect their customers and themselves.
Liveness detection technology was developed as a response to these security demands, initially focusing on passive liveness methods that identify subtle, involuntary cues, like natural reflections or depth in an image, to confirm the presence of a live user. While effective in its time, passive liveness faced limitations against advanced spoofing techniques, such as replayed videos and deepfake technology. In response, active liveness detection evolved, adding a layer of user interaction, such as smiling before the image will be taken.
Now, dynamic liveness detection represents an even more advanced iteration, offering superior security through randomisation requests. By prompting users to perform unpredictable actions—such as specific head movements or facial gestures—this method significantly raises the bar against fraud attempts, making real-time replication or spoofing exceptionally difficult. This interactive layer bolstered security by making it harder for fraudsters to use static images or pre-recorded videos.
What is Liveness Detection?
Liveness detection involves prompting users to perform specific actions during the verification process. These actions prove that the biometric data belongs to a living person. By requiring interaction, the system can confirm the presence of a real individual, not a fake representation, unlike facial recognition, which only confirms if an image is real or not.
Liveness detection is a vital security measure in digital identity verification. It ensures that the person attempting to access a system is alive and present, not using a static image or recording. With the rise of biometric security systems, distinguishing between a live person and a spoof attempt becomes crucial. Liveness detection plays a key role in enhancing security by preventing presentation attacks and building trust in biometric systems.
Liveness detection safeguards against presentation attacks. Fraudsters might use photos or masks to trick systems. This approach helps prevent unauthorised access by validating that the user is truly present.
Several actions can confirm liveness. Users might be asked to:
- Blink: This action is difficult to replicate in a photo.
- Nod: A simple head movement that verifies presence.
- Head rotation: rotational head movements that further verify presence.
The primary goal is to ensure interaction with a live subject which differs from regular facial recognition which just checks if the image is a real face or not. This approach makes it nearly impossible for static images or recordings to deceive the system. As a result, organisations can maintain higher security standards and protect sensitive information effectively.
Liveness Detection Vs Face Recognition
Understanding the distinction between liveness detection and face recognition is crucial. While they are closely related, each serves a unique function in identity verification systems.
While both technologies are essential, liveness detection offers superior security benefits in preventing fraud and ensuring that identity verification systems are not easily bypassed.
- Prevents Spoofing Attacks: Liveness detection is specifically designed to stop common fraud tactics like using photos, videos, or even sophisticated 3D masks to impersonate a user. It ensures that the person presenting the face is physically present and interacting with the system in real time.
- Enhances Security: In a world where deepfake technology and advanced spoofing methods are on the rise, liveness detection adds an extra layer of protection. Without it, face recognition systems could be more vulnerable to fraudulent attempts, compromising overall security.
- Critical for High-Risk Industries: Industries like banking, fintech, and digital payments rely heavily on verifying the identity of individuals. Liveness detection provides the assurance that the person attempting access is real, reducing the risk of fraud in high-stakes scenarios.
- Continuous Improvement: With advancements in machine learning, modern liveness detection systems learn from every fraudulent attempt, becoming smarter over time. This adaptive nature makes liveness detection a constantly evolving safeguard, ensuring it stays ahead of fraudsters.
What is Dynamic Liveness Detection?
Dynamic liveness detection enhances active liveness technology by introducing randomised prompts for users to complete during verification. While active liveness detection already adds an additional security layer over passive methods by requiring specific actions (like a smile, blink, or nod), it’s still potentially vulnerable to sophisticated fraud attempts. Fraudsters can study systems with predictable actions and generate realistic images or videos tailored to mimic these cues in the exact sequence, creating a risk of bypass.
Dynamic liveness detection addresses this by randomising the actions requested from users, removing any fixed sequence that fraudsters could anticipate or exploit. This randomisation ensures that even highly realistic synthetic attempts fail, as they can’t predict the specific prompts, providing a stronger defence against evolving fraud tactics and securing the verification process more effectively.
Why Dynamic Liveness Detection is Crucial for Security
Here’s why dynamic liveness detection is a transformative asset for security:
a. Advanced Protection Against Fraud
Dynamic liveness detection enhances security by making it significantly harder for fraudsters to bypass. Unlike traditional active liveness, which prompts users to perform set sequenced actions like blinking or nodding, dynamic liveness introduces a random sequence of actions. This unpredictability creates a strong defence, as fraudsters cannot anticipate the specific actions needed. By preventing attackers from programming deepfakes or synthetic images to mimic a fixed sequence, dynamic liveness detection offers unparalleled protection against identity theft, spoofing, and unauthorised access.
b. Increased User Trust in Biometric Systems
Biometric systems thrive on user trust, and dynamic liveness detection strengthens this confidence by ensuring only real, live individuals can complete the verification process. When users know that their biometric data is safeguarded by randomised actions, they feel more secure and are more likely to trust and adopt these systems.
c. Enhanced Compliance with Regulatory Standards
Dynamic liveness detection also supports compliance with regulatory standards, especially in industries where data protection and security are paramount, like finance, healthcare, and government sectors. Many regulations require robust KYC measures to protect personal information from unauthorised access. By implementing advanced dynamic liveness detection, organisations can meet these standards and offer the highest security for user data, ensuring they are compliant with legal requirements for data protection and security.
d. Versatile Applications Across Industries
The versatility of dynamic liveness detection makes it suitable across various sectors. In banking, it safeguards accounts by ensuring that the person making a transaction is genuine. In telecommunications, it prevents account takeovers, and in remote onboarding, it verifies users securely from any location. The adaptability of dynamic liveness allows businesses to integrate highly secure, user-friendly verification processes tailored to industry needs, providing comprehensive security across different applications.
What are the Different Types of Liveness Detection?
Understanding the types of liveness detection helps in choosing the right security approach for various applications. There are two main types of liveness detection: passive and active, with some systems using hybrid or dynamic approaches. Each method offers distinct features and benefits:
1. Passive Liveness Detection
Unlike active methods, passive liveness detection operates seamlessly in the background, requiring no direct interaction from the user. It relies on analysing natural biometric traits such as skin texture, blood flow, or subtle facial micro-movements to verify the presence of a live individual. This approach enhances the user experience by providing an uninterrupted and smooth verification process, which is ideal for applications where minimal friction is desired. Despite its less direct approach, passive liveness detection effectively confirms liveness by examining biometric details that are hard to replicate, making it a valuable layer of security in scenarios where user convenience is prioritised.
2. Active Liveness Detection
Active liveness detection involves direct interaction with the user, requiring specific actions like head movement, blinking, or smiling to verify their presence. This form of liveness detection operates by demanding real-time responses, making it highly resistant to fraudulent attacks using static images or pre-recorded videos. Because active liveness checks for unique, live responses, it offers a high level of accuracy and is particularly effective against basic spoofing attempts.
3. Hybrid or Dynamic Liveness Detection
Hybrid approaches combine active and passive techniques, leveraging the benefits of each to provide robust, layered security. By integrating active liveness methods—including dynamic liveness detection—alongside passive checks, hybrid models deliver an enhanced defence against sophisticated spoofing attempts. The dynamic component allows for randomised prompts, making it nearly impossible for fraudsters to replicate the verification process. This adaptability makes hybrid systems suitable for varied applications, where organisations can customise the level of security based on specific needs.
Passive vs Active vs Dynamic Liveness Detection
Here's a comparative table that outlines the differences between passive, active, and dynamic liveness detection:
Types of Presentation Attacks and Recommended Solutions to Combat Them
Presentation attacks, often called spoofing attacks, are deliberate attempts by malicious actors to deceive biometric systems into granting unauthorised access. Key types of presentation attacks include:
i. Print Attacks
In print attacks, fraudsters use printed images, such as high-resolution photos, to impersonate a legitimate user. These images are designed to closely replicate the user's facial features to deceive basic biometric systems. While these attacks are typically low-cost and relatively unsophisticated, they can be effective against systems that lack advanced liveness detection.
Print attacks can be countered by employing passive liveness detection, which scans for biometric signals such as subtle skin texture and facial depth that are absent in flat images.
ii. Replay Attacks
Replay attacks involve using pre-recorded videos or photos to mimic a legitimate user. Attackers often gather videos from social media or other online sources to create a lifelike impression that can fool systems lacking dynamic liveness features. These attacks are more complex than print attacks because the video allows for dynamic movement that closely resembles a live user.
However, replay attacks are thwarted by active and dynamic liveness detection methods, as these methods require specific user interactions that a pre-recorded video cannot accurately reproduce.
iii. Mask Attacks
Mask attacks are more advanced and involve attackers wearing a 3D mask or facial replica of the legitimate user. The mask is created using high-quality materials to simulate human features like skin texture, colour, and even reflections, making it challenging for standard verification systems to detect.
Since these attacks introduce realistic depth and texture, systems relying on basic or passive liveness detection only are often at risk. Dynamic liveness detection is particularly effective here, as the randomised prompts are challenging to replicate with a static mask.
iv. 3D Model Attacks
In 3D model attacks, attackers create sophisticated replicas using 3D printers, sculpted models, or advanced rendering techniques. These replicas can mimic the depth, contours, and detailed features of a legitimate user’s face, making them difficult to detect without advanced biometric security.
Dynamic liveness detection is highly effective in this case, as its randomised prompts can disrupt attempts by 3D models to simulate a live response. The high cost and technical expertise required for 3D model attacks make them rare but particularly dangerous.
v. Synthetic Media Attacks (Deepfakes)
Synthetic media attacks, commonly referred to as deepfakes, use AI and machine learning to create highly realistic videos or images that mimic a person’s face and expressions. Attackers can leverage this technology to generate believable but entirely synthetic biometric data, which poses a serious threat to systems without robust liveness detection.
Deepfake attacks are among the most complex and can bypass traditional systems by replicating realistic expressions and micro-movements. However, dynamic liveness detection, with its randomised and unpredictable prompts, adds a critical layer of security, as it requires spontaneous actions that deepfake algorithms cannot easily replicate in real-time.
Why Dynamic Liveness Detection is the Future of User Onboarding
Dynamic liveness detection is set to transform user onboarding, making it more secure and user-friendly. Here's why it holds the future:
a. Advanced AI Integration
With dynamic liveness detection, AI algorithms play a critical role, enabling the system to detect and adapt to even the most advanced spoofing attempts. These AI-driven algorithms continuously learn from new data, which allows them to stay ahead of emerging fraud techniques and ensures precise differentiation between genuine users and sophisticated forgeries. By continuously updating, the system keeps security strong, intelligently adjusting to potential threats and bolstering trust for end-users and organisations alike.
b. Dynamic Verification
Dynamic liveness detection takes active liveness a step further by incorporating randomised, real-time prompts that make it exceedingly difficult for fraudsters to predict or bypass. This dynamic interaction could involve a combination of gestures, like nodding or performing randomised facial movements. By requiring both actions and responses that change unpredictably, dynamic liveness detection ensures that only real, present users can complete the verification process, creating a strong defence against spoofing methods that rely on scripted, predictable behaviours.
c. Wide Adoption Across Industries
The growing prevalence of dynamic liveness detection highlights its critical role in high-stakes sectors. The financial industry, particularly fintech, is adopting this technology to secure user accounts and safeguard sensitive transactions, mitigating the risks posed by identity theft. Governments are also implementing this advanced liveness detection as they move to secure digital identity and citizen verification, ensuring only authorised individuals can access services.
d. Future Innovations in User Experience
The future of dynamic liveness detection includes potential integrations with augmented reality (AR), which could guide users through the onboarding process using virtual cues, making verification interactive and intuitive. Picture a scenario where a virtual assistant provides real-time instructions within an AR interface, directing users to perform specific actions that secure their verification while creating a more engaging user experience.
Achieving Optimal User Security with Smile ID Dynamic Liveness Detection
Active liveness detection has become a critical tool in the fight against fraud, particularly in identity verification processes. Unlike face recognition, which identifies or verifies an individual’s identity, liveness detection ensures that the biometric data being provided comes from a real, live person. This combination of technologies provides a strong, multi-layered security system that enhances both accuracy and trust in remote authentication. As organisations increasingly rely on digital KYC processes, liveness detection helps safeguard against fraudulent attempts using static images, pre-recorded videos, or sophisticated spoofs like 3D masks.
At Smile ID, we have taken liveness detection to the next level with our dynamic liveness feature we’ve called Enhanced SmartSelfie™ which is available across our biometric authentication suite [hyperlink]. Having passed the ISO/IEC 30107-3:2023 certification with 0% facial fraud attack penetration, this unique feature ensures ongoing user security, which is particularly important for high-risk industries such as banking, remittance, digital merchant acquiring, and payments.
Key Features of Smile ID’s Enhanced SmartSelfie™
- Dynamic Randomised Liveness Challenges: Users are now asked to perform random actions like head movements or facial gestures, making it significantly harder for fraud attempts.
- Enhanced Selfie Quality Analysis: A new quality scoring system (0-100 scale) ensures selfies meet high standards, rejecting low-quality images that may indicate fraud.
- Advanced Fraud Detection Algorithms: Increased metadata collection and real-time fraud analysis provide deeper insights into user behaviour and suspicious activities.
- Real-Time UI Feedback: Instant feedback helps users easily meet verification requirements, reducing frustration and improving the user experience.
- Strict Mode for Compliance: Essential for high-security environments, this mode enforces rigorous image capture standards.
See Enhanced Smartselfie in action 👇
Smile ID’s Biometric Solution Suite
Our focused biometric authentication suite - SmartSelfie Enrollment, SmartSelfie Authentication, SmartSelfie Compare, Smile Secure, Biometric KYC and Document Verification - now leverages dynamic liveness technology (Enhanced SmartSelfie), each offering unique capabilities to enhance security and streamline user onboarding and ongoing monitoring processes.
With Smile ID, businesses can remain confident in their user verification processes while staying compliant with global regulations. Get started today, book a free demo to see how our solution can automate your KYC process and save you time and resources.
Ready to get started?
We are equipped to help you level up your KYC/AML compliance stack. Our team is ready to understand your needs, answer questions, and set up your account.